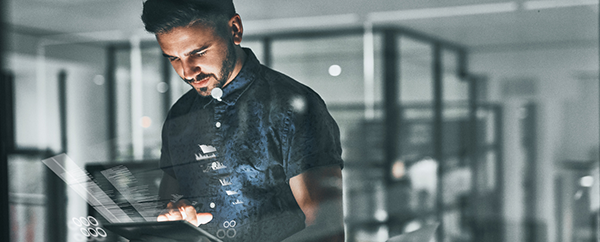
How our Data Science team and ACM built SubroFy, an innovative subrogation tool
- When ACM wanted to identify more subrogation opportunities, they called on Arrowhead Programs’ Data Science team.
- Working together, they created a list of keywords from closed subrogated claims to use as identifiers for potential high opportunity claims for subrogation.
- Using machine learning and natural language processing, the subrogation tool was created, uncovering subrogation opportunities in substantially more claims.
A great claims administrator not only resolves claims quickly and accurately – they look for hidden subrogation opportunities to mitigate loss experience. Often those successful subrogation cases result in significant savings for carriers.
American Claims Management (ACM), one of our Arrowhead Programs’ services companies, worked with our Data Science & Analytics team to create a state-of-the-art subrogation tool called SubroFy. We met recently with ACM’s President Dhara Patel, along with Daren Eiri (director of Data Science) and Jason Perone (data scientist) to learn more about this first-of-its-kind subrogation tool, and how it was built.
Related: How our Data Science & Analytics team helps companies boost their bottom line
What were the challenges you faced that made you decide to call in the Data Science & Analytics team?
Dhara: While we had used traditional subrogation triggers in the past, we needed to develop a more sophisticated approach to data to find opportunities that wouldn’t otherwise be identified.
In the claims industry, subrogation is probably one of the areas that has the highest amount of missed opportunities. There’s a lot of hidden subrogation potential that may not be obvious to even the most experienced adjuster. We needed the Data Science team to look at our data and help us find more subrogation opportunities.
I think this will be a huge, especially for those that either outsource their claims or even have their own claims department, because I honestly don’t know of another subrogation tool like this out there.
How did the team get you to where you needed to be?
Dhara: Using our existing data sets, the Data Science team built a tool that uses that data more intelligently. Just as importantly, the resultant tool is user-friendly and fits into our team’s daily workflow in an efficient, focused way, ensuring that it will make a real impact.
Jason: We use an advanced machine learning algorithm tool, coupled with NLP – natural language processing. A big part of identifying subrogation opportunities is the loss description from the adjuster’s notes. NLP takes that loss description from either the adjuster or the CSR and breaks apart the text into individual words. We identified a set of keywords that the tool searches for, based on historically successful subrogation recoveries. Our proprietary subrogation tool then extracts those keywords from a new claim to point out subrogation opportunities. We’re taking specific aspects of the claim and unstructured, free form text to assist in recovering claim dollars for our carrier partners.
Related: How a custom submission grader helps Lawyer’s Protector Plan increase profitability
What’s an example of those keywords the tool searches for?
Jason: One example would be a loss description for a homeowners claim that says a third-party vehicle hit the insured’s home property. In that case, the insurance company would pay to repair damages, then subrogate the claim expense to the vehicle owner’s insurance.
Dhara: Subrogation opportunities like this occur very frequently. It’s why we think there’s a lot of hidden opportunities, because so much of the information that the adjusters are collecting is just sitting, unused, in free form text on their tablets.
Using machine learning coupled with NLP provided by the Data Science & Analytics team helps us capture significantly more of these opportunities. In the future, we can look at a carrier’s loss history along with their loss descriptions and notes and then run a model to see if there are opportunities for savings that they’ve not seen. In other words, we could provide them with the claims that have a definite potential to subrogate.
Some carriers are really, really savvy about subrogation, with a large, dedicated department. They spend a lot of money on it. Other carriers don’t invest their dollars there. Everyone wants subrogation, but few actually build subrogation into their modeling, and I think it simply gets overlooked quite a bit.
Describe the process of building the subrogation tool and how it works
Jason: It was very much a collaborative effort all along the way. We examined the data available in their databases, pulled the data that we needed, cleaned and prepared it for modeling and built a machine learning model. After discussing with ACM as to how they needed the tool to be implemented, we created a process to automatically pull in new claims every single day and grade them in terms of high, medium or low subrogation chance.
For claims that we predicted would have high likelihood of subrogation opportunities, we built a web application that ACM’s claims team could review daily as part of their claims review workflow. They also receive a daily email with new claims that rate high for subrogation possibilities. The good news is that we’ve been able to weed out most of the false positives so the team can focus in on where the greatest possibilities lie.
Daren: This project highlights the importance of the partnership of our Arrowhead Programs companies and the Data Science team. In this case, our partner was ACM. We can’t build a successful product without a strong client partnership in a highly collaborative environment. That’s what ultimately makes it successful.
Dhara: I agree. It’s been a great experience in terms of collaborating and learning from the Data Science team, while sharing our claims expertise to help drive the details of the model within the tool. We’re excited to be able to use data in innovative ways to make more intelligent decisions – in a more automated fashion – producing positive results that favorably impact an insurer’s bottom line.
These subrogation opportunities are hidden-value impact areas to a loss ratio. An actuary may build in a certain amount of subrogation, but often there’s not enough of an emphasis, versus how powerful a subrogation can be on a loss ratio. In a way, it’s found money, right?
We’re not just looking at data. We’re getting into the why of the subrogation: The potential for how it impacts the claim paid and therefore the company’s bottom line.
We embarked on a powerful partnership with our internal Data Science team using machine learning to create a unique subrogation model. We believe this subrogation tool is going to produce unexpectedly positive results on loss ratios for all of our clients going forward, taking our claims services to the next level.